Cobweb @Vision Task
A study about forgetting effects in Cobweb model in vision tasks.
Cobweb represents a human-like learning system specifically designed for conceptual learning through examples or instances. It arranges concepts in a hierarchical tree structure using a top-down classification approach while continuously acquiring new knowledge.
In our study, we introduce an adapted version of the Cobweb model optimized for processing image data within the tree framework. Our findings demonstrate that this model significantly mitigates the issue of catastrophic forgetting in comparison to neural networks. Moreover, the model showcases enhanced resilience in concept acquisition from visual input, illustrating its ability to learn visual concepts in a modular manner rather than focusing solely on pixel-level information and data scale dependence inherent in neural networks.
Great thanks to all the collaborators and people giving critiques for this project: Nicki Barari (Drexel), Chris MacLellan (GaTech), Jesse Roberts (Vanderbilt), Doug Fisher (Vanderbilt)
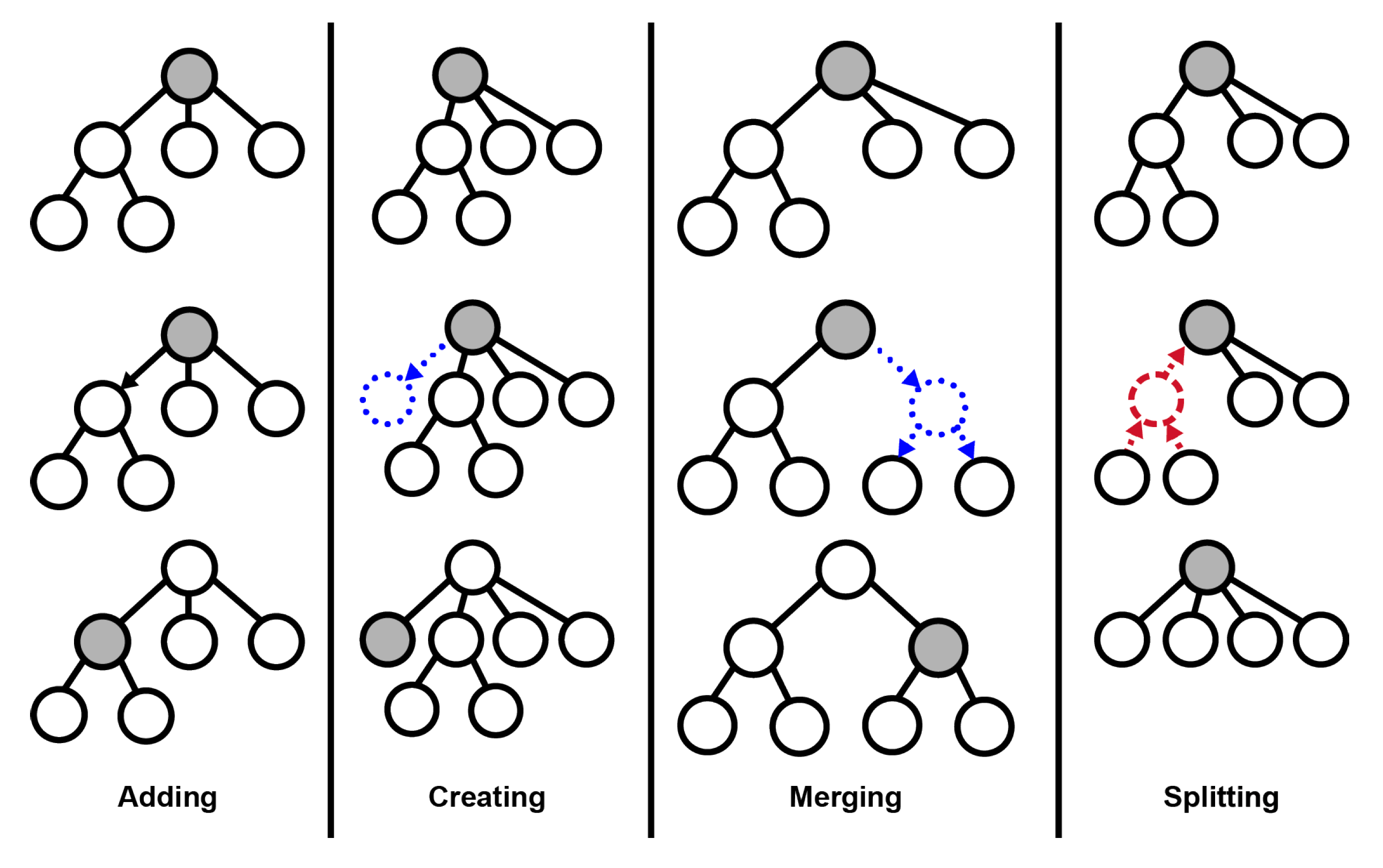